Predictive analytics uses historical data (concerning transactions and website visits), statistical algorithms, and machine learning (AI) technology to estimate future results and predict future events. In SALESmanago, prediction values are provided for both individual Contacts and your entire database.
This article describes Predictive Analytics for individual Contacts, which is presented on their Contact Cards under the Predictive Analytics tab. To view collective predictions for your entire database, visit the Predictive Analytics Dashboard.
Learn more about the Predictive Analytics Dashboard >>
The data presented in the Predictive Analytics tab on the Contact Card enables you to identify current trends in the behavior of individual Contacts and adapt your marketing communications accordingly, for example, to help your customers make the purchase decision or encourage them to come back to your e-store.
Learn more about Predictive Analytics in SALESmanago and how to apply it in practice >>
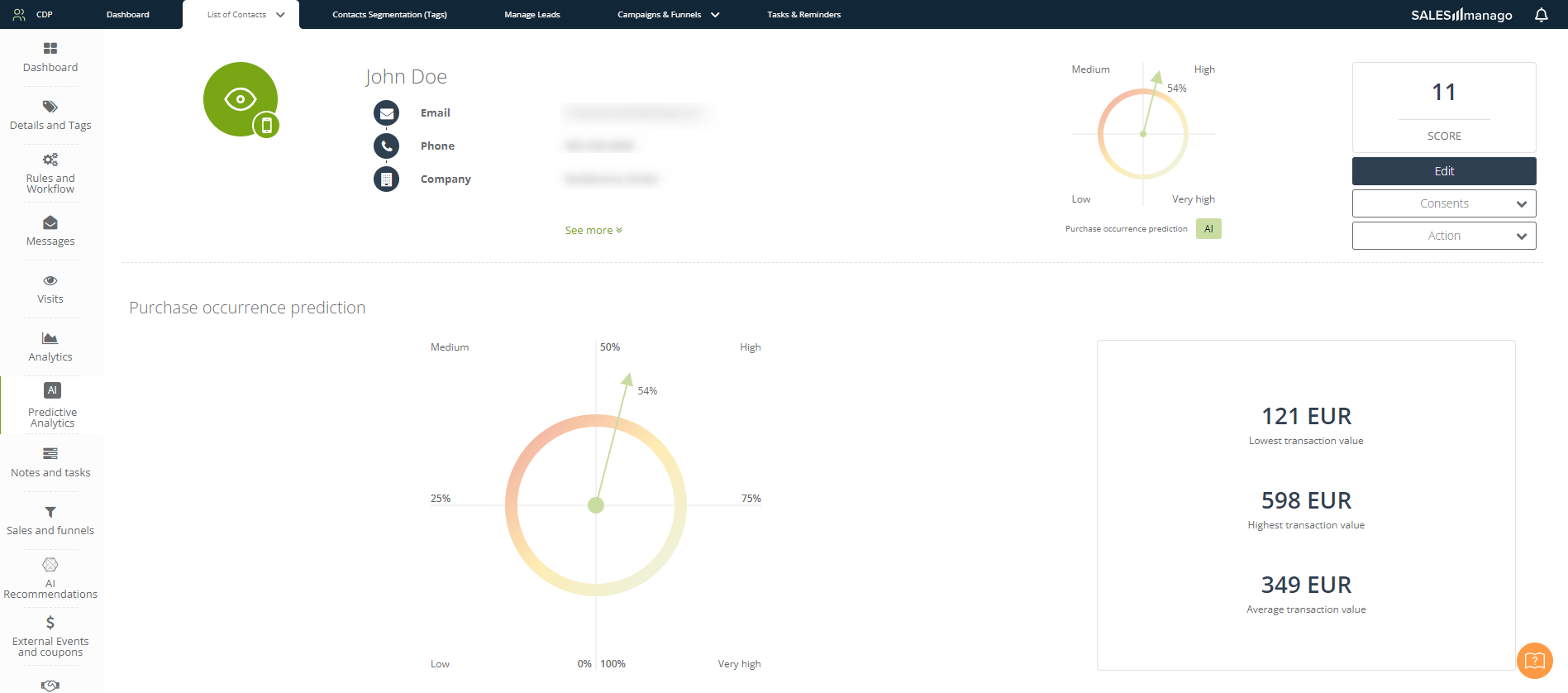
To view Predictive Analytics for an individual Contact, go to
Audiences → Contacts → Contact management → Contact List → Contact Card → Predictive Analytics tab
NOTE:This feature is not available in all SALESmanago plans. If you are interested in this add-on, please contact your Customer Success Manager.
Contents:
- Getting started
- Purchase occurrence prediction
- Customer Lifetime Value (CLV) analysis and churn prediction
- Attribution prediction
1. Getting started
Predictive analytics involves using historical data and machine learning algorithms to identify patterns and trends within existing data and, on this basis, make predictions about future events.
In SALESmanago, you can see different types of predictions for each individual Contact by going to Menu → Audiences → Contacts → Contact management → Contact List and selecting the Contact you are interested in. Once you are on their Contact Card, go to the Prediction Analytics tab.
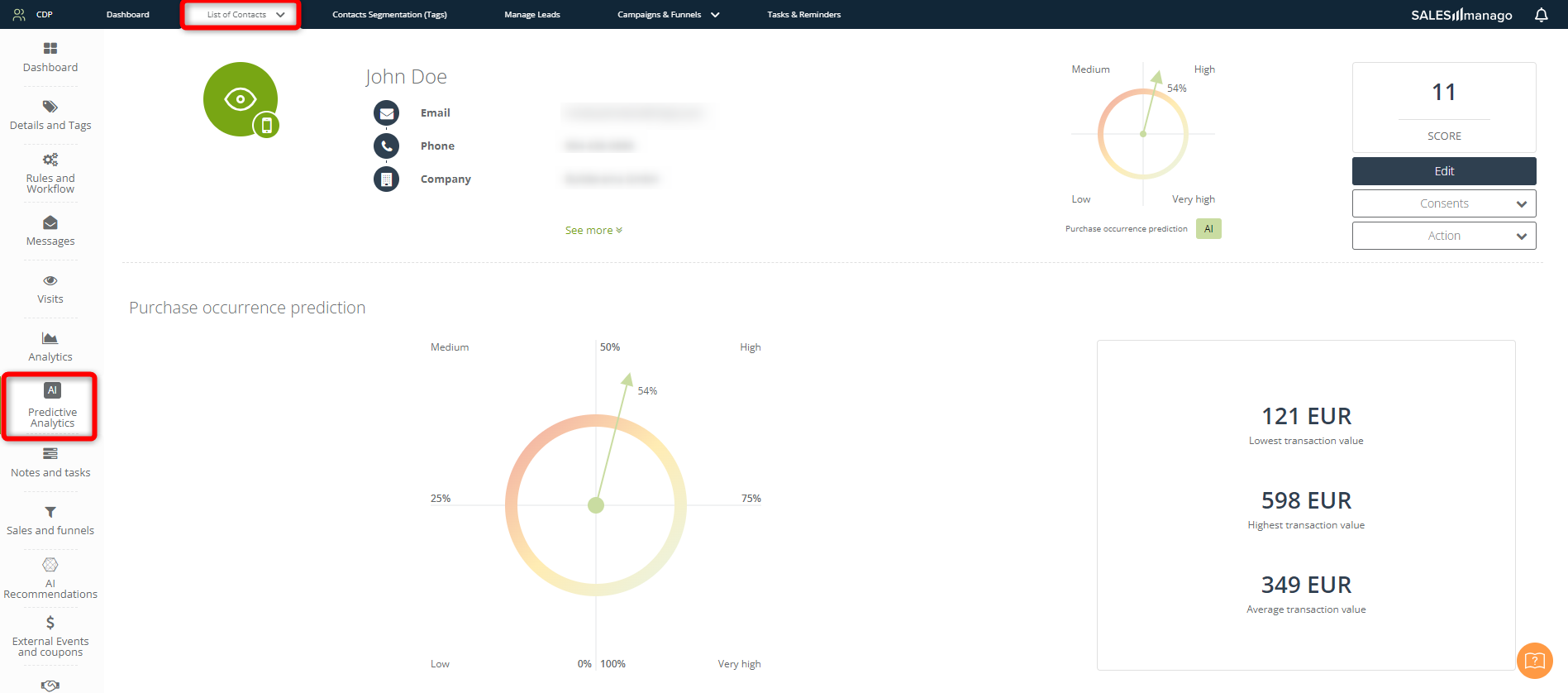
The contents of this tab are divided into three sections:
- Purchase occurrence prediction—How likely it is that the Contact will make a purchase.
- Customer Lifetime Value (CLV) and churn prediction—The estimated CLV of this Contact, basic information about their purchase history, and how likely it is that the Contact will churn (i.e., stop being your customer).
- Attribution—How likely it is that different channels will contribute to the Contact’s purchase decision.
Read on for a detailed description of each of these sections.
To learn how you can apply this data in practice, read the article about Predictive Analytics in SALESmanago >>
2. Purchase occurrence prediction
This section presents the purchase prediction value for the selected Contact, calculated based on the Contact’s transactional data and their visits to your website.
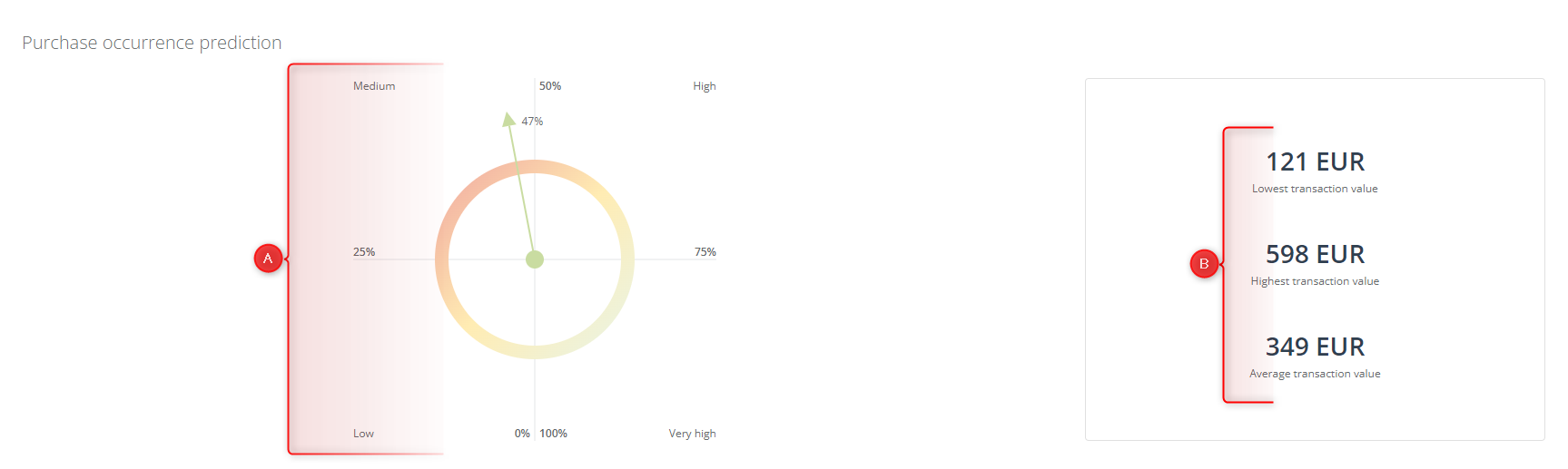
The arrow on the chart [A] indicates the purchase prediction value for this individual Contact. The value can fall into one of the following ranges:
- Low—between 0 and 25%—if the Contact is unlikely to make a purchase,
- Medium—between 25% to 50%—if the Contact is not very likely to but might make a purchase,
- High—between 50% to 75%—if the Contact is likely to make a purchase,
- Very high—between 75% to 100%—if the Contact is very likely to make a purchase.
Note that whichever Contact Card tab you visit, you can always see a miniature version of this chart next to the Contact’s basic information:

To the right of the chart, you can see important transactional information about this specific Contact [B]:
- Lowest transaction value—The smallest amount of money the Contact has spent on a single transaction so far.
- Highest transaction value—The largest amount of money the Contact has spent on a single transaction so far.
- Average transaction value—The average amount of money the Contact has spent on their transactions so far.
3. Customer Lifetime Value (CLV) analysis and churn prediction
Here, you can see the Customer Lifetime Value (CLV) analysis for an individual Contact, their churn prediction value, and detailed information concerning their transactional history.
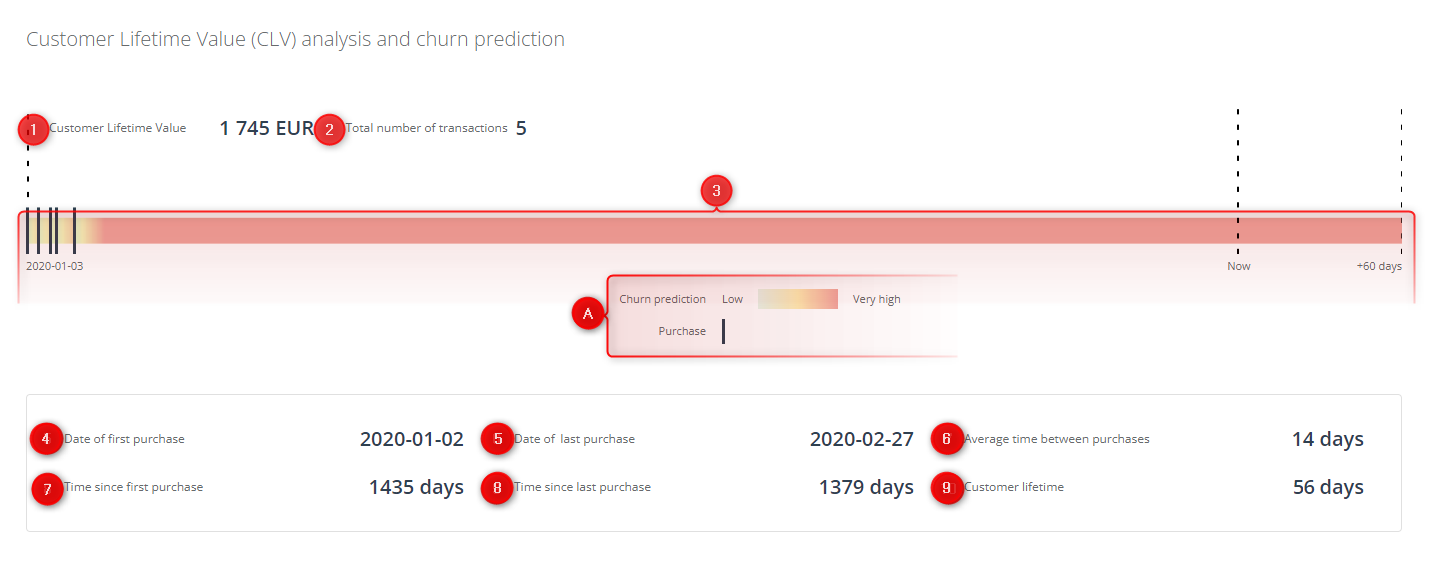
[1] Customer Lifetime Value—Here, you can see the total amount of money this Contact has spent in your store to date.
[2] Total number of transactions—Here, you can see the total number of purchases this Contact has made in your store to date.
[3] Chart—Here, you can see churn prediction data for this Contact, including:
- a visualization of historical data showing how the Contact’s churn prediction value changed in the past,
- the current likelihood of churn,
- the likelihood of churn in the next 60 days.
[A] Legend—Here, you can see which colors correspond to the particular ranges of churn prediction values and how purchase occurrences are indicated on the chart.
[4] Date of first purchase—Here, you can see when the Contact made their first purchase in your store.
[5] Date of last purchase—Here, you can see when the Contact made their most recent purchase in your store.
[6] Average time between purchases—Here, you can see the average number of days that pass between two consecutive purchases made by this Contact. This value allows you to estimate when the Contact is likely to make a purchase again.
[7] Time since first purchase—Here, you can see the number of days that have passed between the Contact’s first purchase in your store and the present moment.
[8] Time since last purchase—Here, you can see the number of days that have passed between the Contact’s most recent purchase in your store and the present moment.
[9] Customer lifetime—Here, you can see the number of days that have passed between the Contact’s first and last purchase in your store.
4. Attribution prediction
This section shows how likely it is that your individual marketing channels will contribute to the purchase decision of an individual Contact (as a touchpoint). The “spider’s web” you can see in this section includes most marketing channels (or their subtypes) that can be used and managed via SALESmanago. The data presented is based on the Contact’s transactional history and website visits.
To determine the probability for a specific channel, locate it on the diagram and check the color of the corresponding dot. Next, consult the legend [A] to determine the prediction value (low, medium, high, very high) associated with that specific channel.
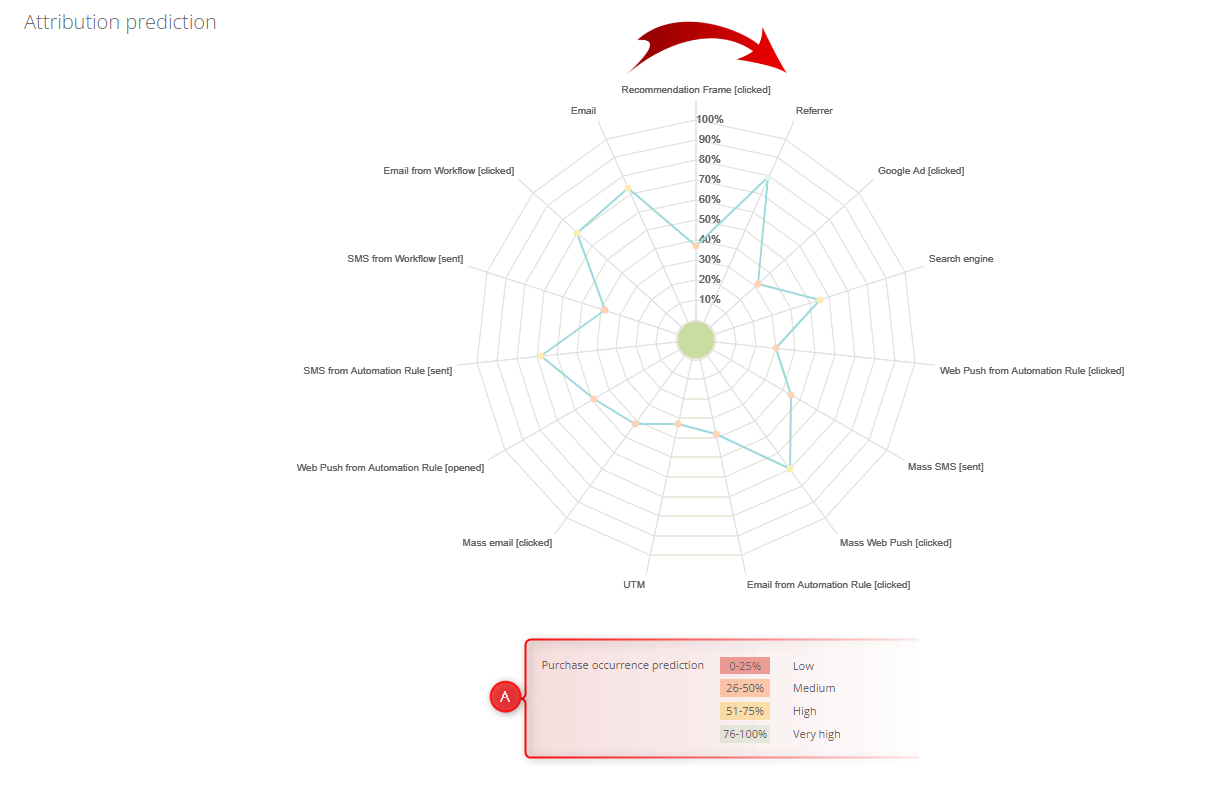
The channels included in the diagram are described below, going clockwise from the top:
- Recommendation frame [clicked]—Here, you can see how likely it is that this Contact will make a purchase if they click on a Recommendation Frame created in SALESmanago and displayed on your website.
- Referrer—Here, you can see how likely it is that this Contact will make a purchase if they visit your website after clicking a link on another (external) website.
Note that two types of links leading to your website are excluded from the Referrer category and presented separately: Google Ads and Search engines.
- Google Ad [clicked]—Here, you can see how likely it is that this Contact will make a purchase if they visit your website after clicking on a Google Ad. A source is considered a Google Ad if it meets one of the following conditions:
- the referrer is google.com AND the target (destination) URL contains /aclk/,
- the referrer is g.doubleclick.net,
- the visited URL on your website includes /gclid/.
- Search engine—Here, you can see how likely it is that this Contact will make a purchase if they visit your website after clicking a result in a search engine (SERP).
See the list of search engines supported by SALESmanago’s attribution system >>
- Web Push from Automation Rule [clicked]—Here, you can see how likely it is that this Contact will make a purchase if they click a Web Push notification created in SALESmanago and sent as part of an Automation Rule.
- Mass SMS [sent]—Here, you can see how likely it is that this Contact will make a purchase if they receive an SMS message sent via SALESmanago as a mass communication.
- Mass Web Push [clicked]—Here, you can see how likely it is that this Contact will make a purchase if they click a Web Push notification created in SALESmanago and sent as a mass notification.
- Email from Automation Rule [clicked]—Here, you can see how likely it is that this Contact will make a purchase if they click a link in an email created in SALESmanago and sent as part of an Automation Rule.
- UTM—Here, you can see how likely it is that this Contact will make a purchase if they visit your website after clicking a link containing a UTM parameter.
- Mass email [clicked]—Here, you can see how likely it is that this Contact will make a purchase if they click a link in an email created in SALESmanago and sent as a mass mailing.
- Web Push from Automation Rule [opened]—Here, you can see how likely it is that this Contact will make a purchase if a Web Push notification created in SALESmanago and sent as part of an Automation Rule is displayed to them.
- SMS from Automation Rule [sent]—Here, you can see how likely it is that this Contact will make a purchase if they receive an SMS message sent as part of an Automation Rule.
- SMS from Workflow [sent]—Here, you can see how likely it is that this Contact will make a purchase if they receive an SMS message sent as part of a Workflow.
- Email from Workflow [clicked]—Here, you can see how likely it is that this Contact will make a purchase if they click a link in an email created in SALESmanago and sent as part of a Workflow.
[A] Legend—Here, you can see which percentages and colors correspond to the particular prediction value ranges.